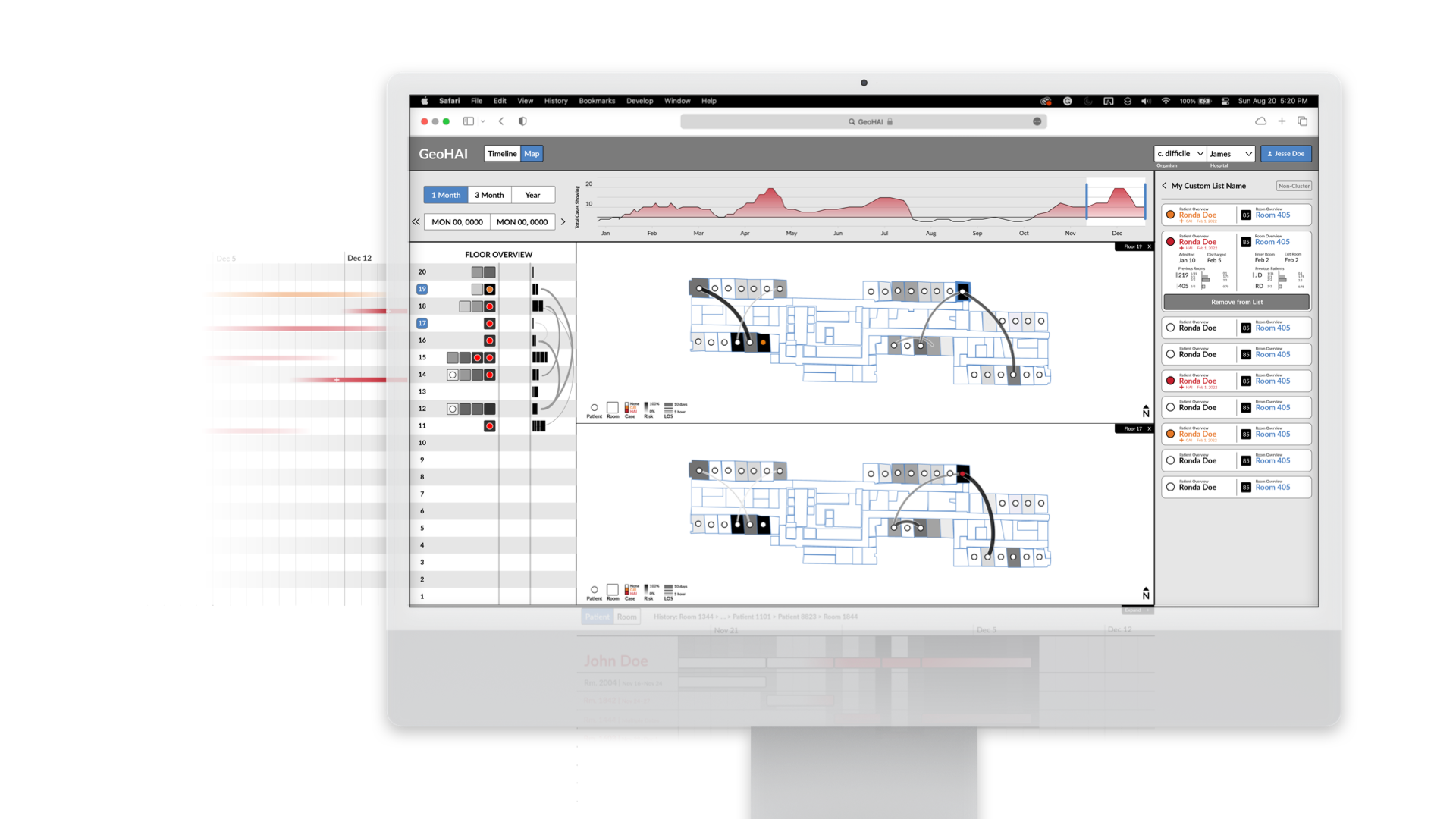
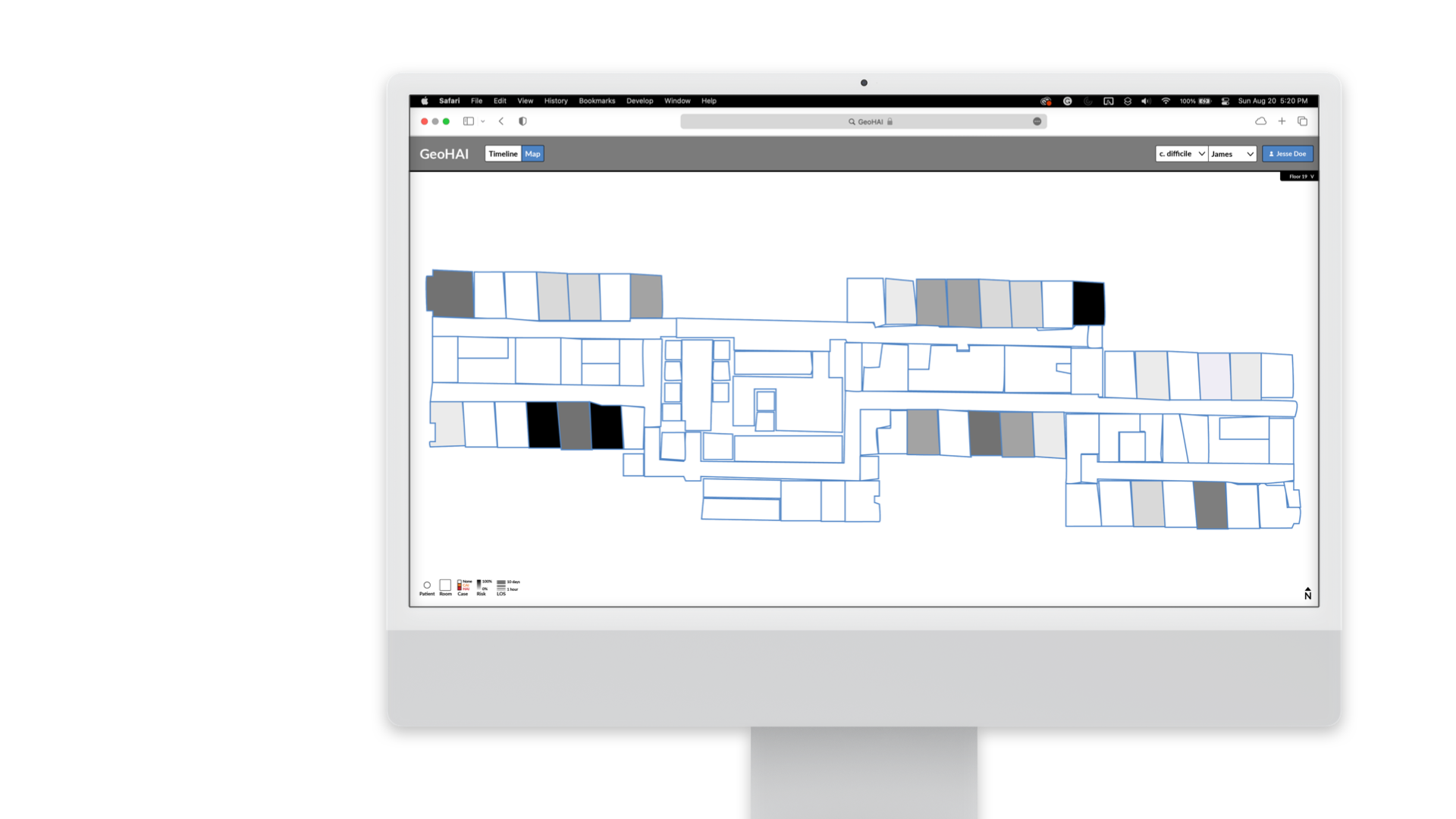
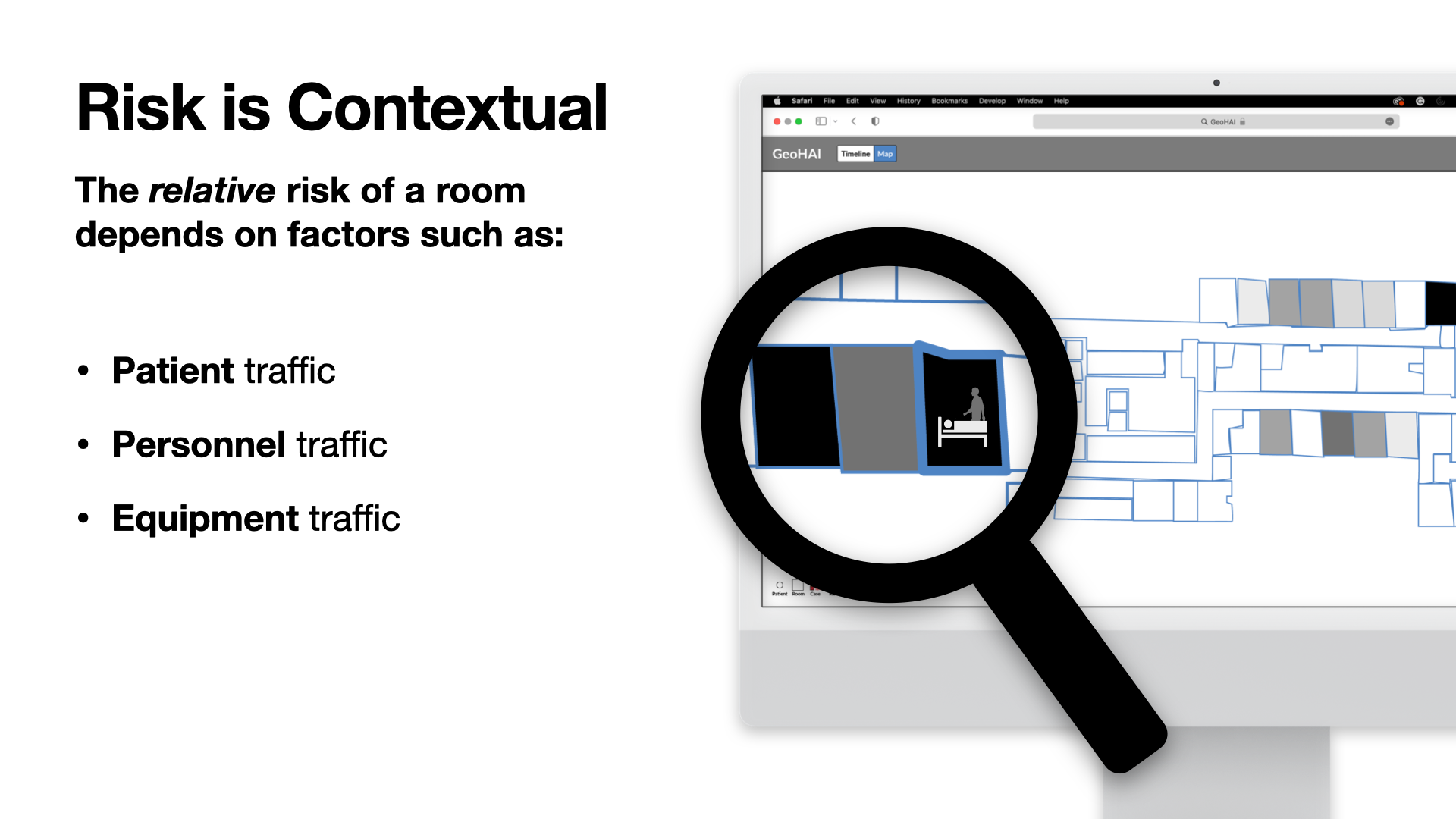
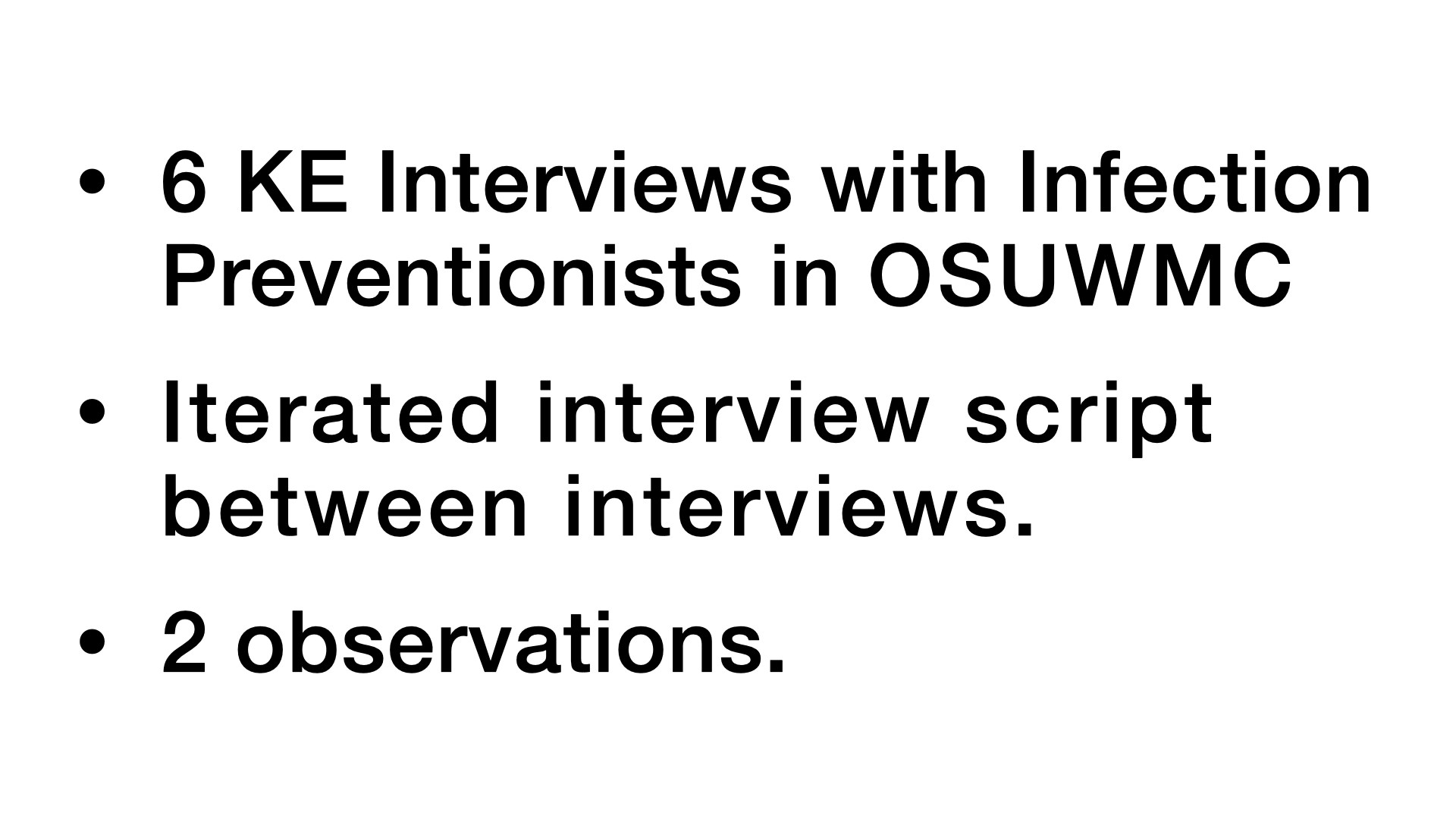
We conducted knowledge-elicitation interviews and observations in Ohio State's university hospital (OSUWMC). Our script was iterated across sessions as interviews led to more questions or revealed discrepancies across units.
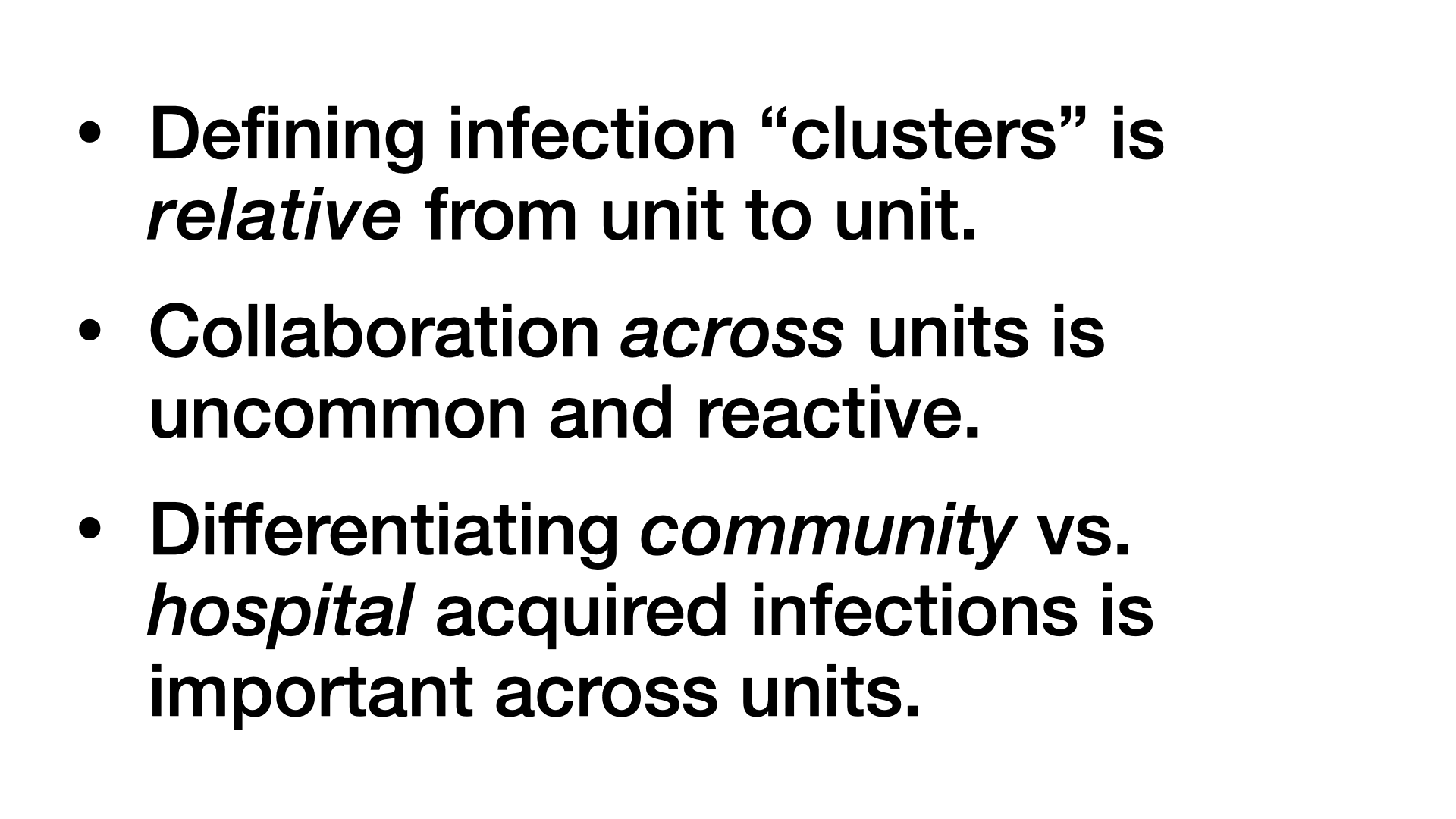
We found the definition of infection “clusters”—the term for a group of related cases—differed from unit to unit, current tooling did not provide shared perspectives across units, and differentiating CAI/HAI was a vital reference for the work context, despite its insignificance to the risk of infection transmission.
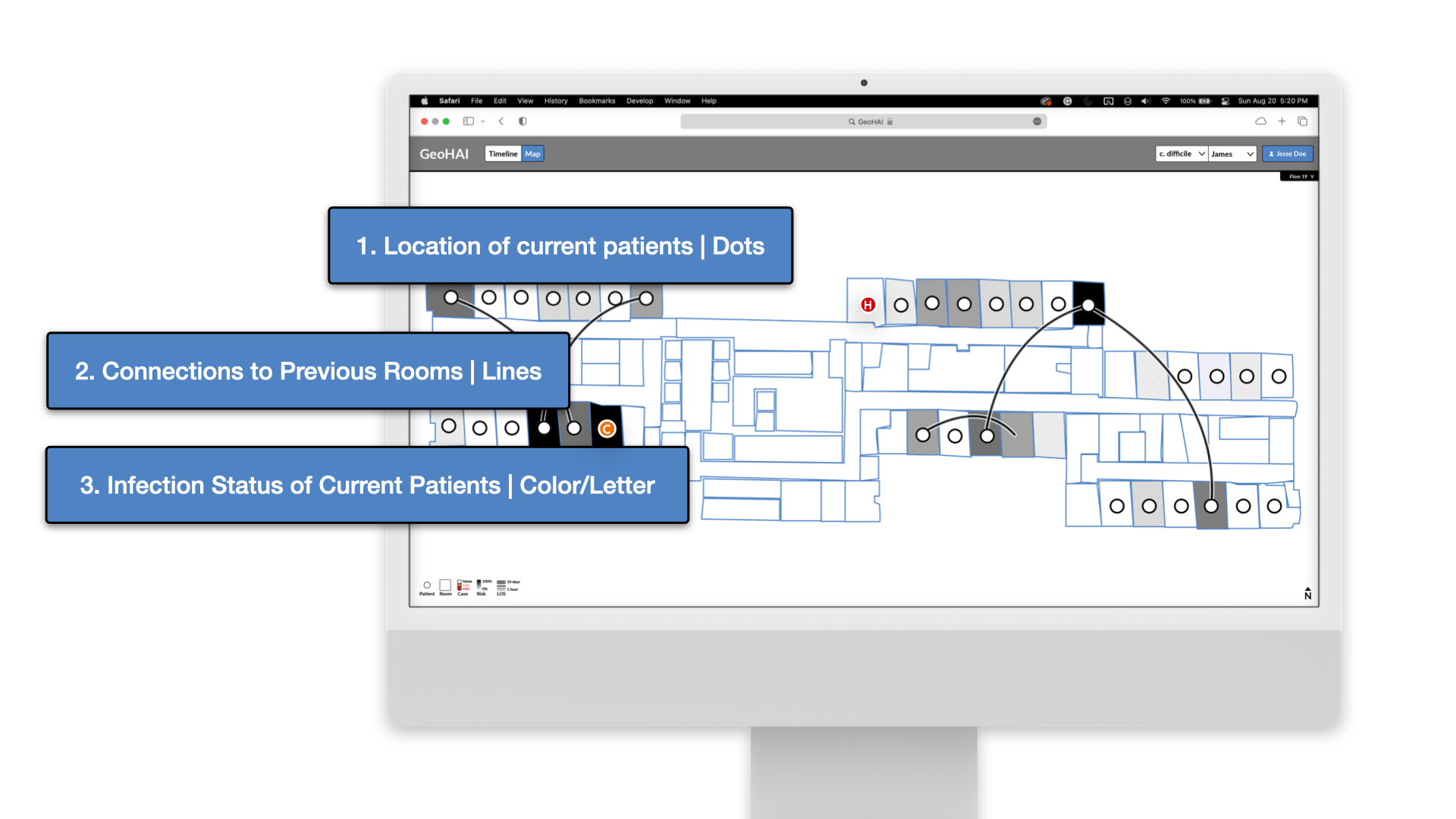
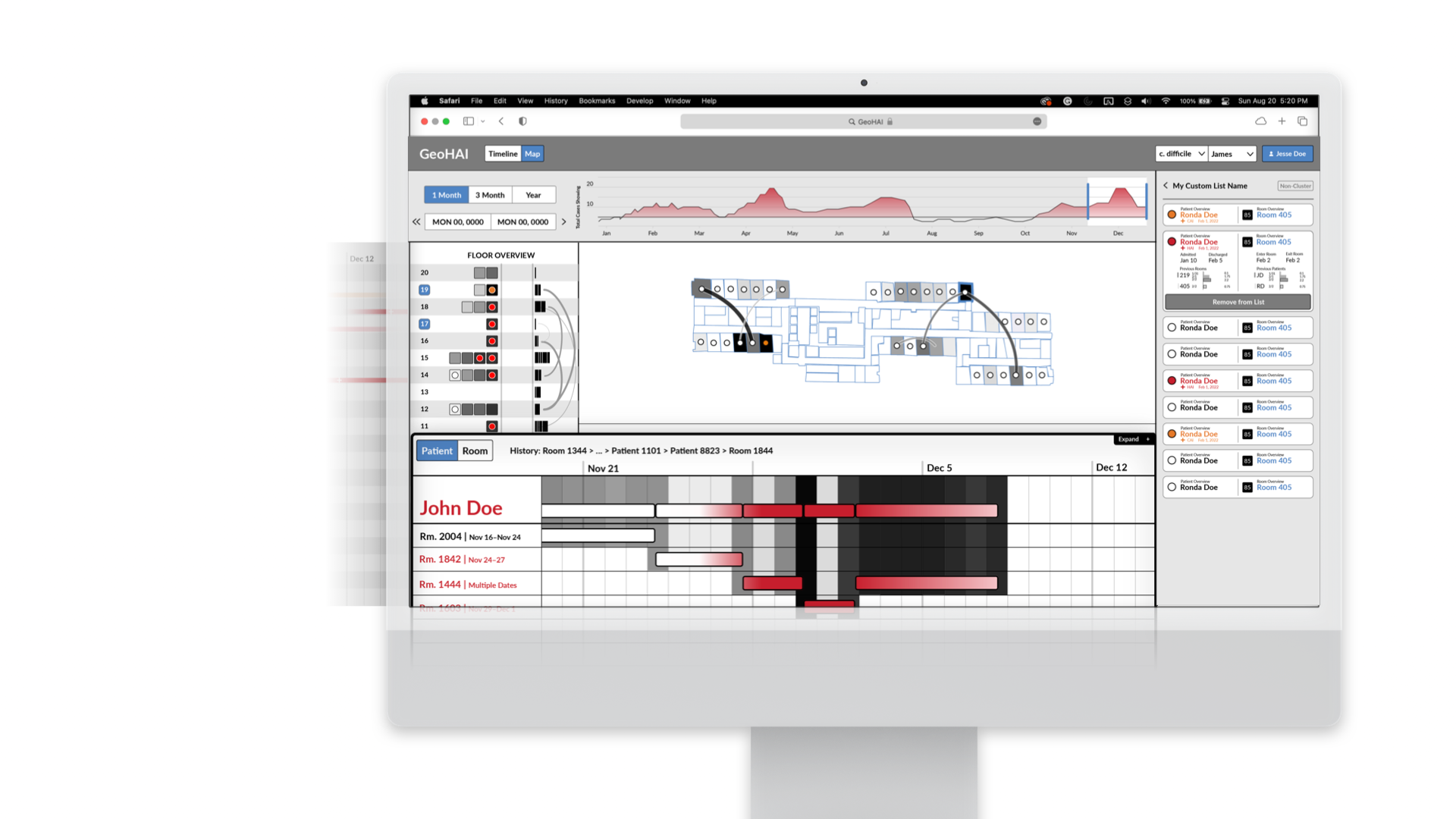
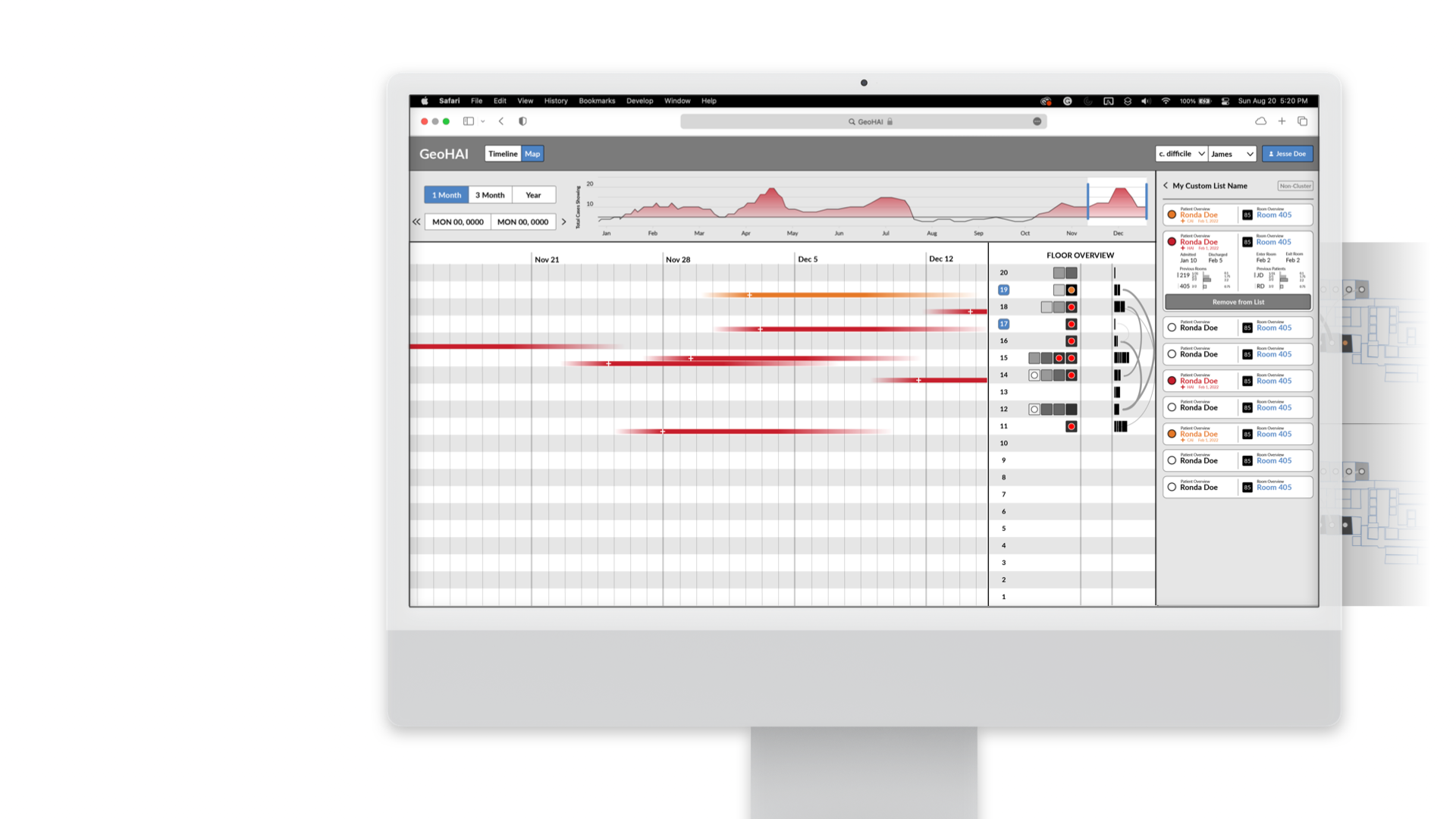
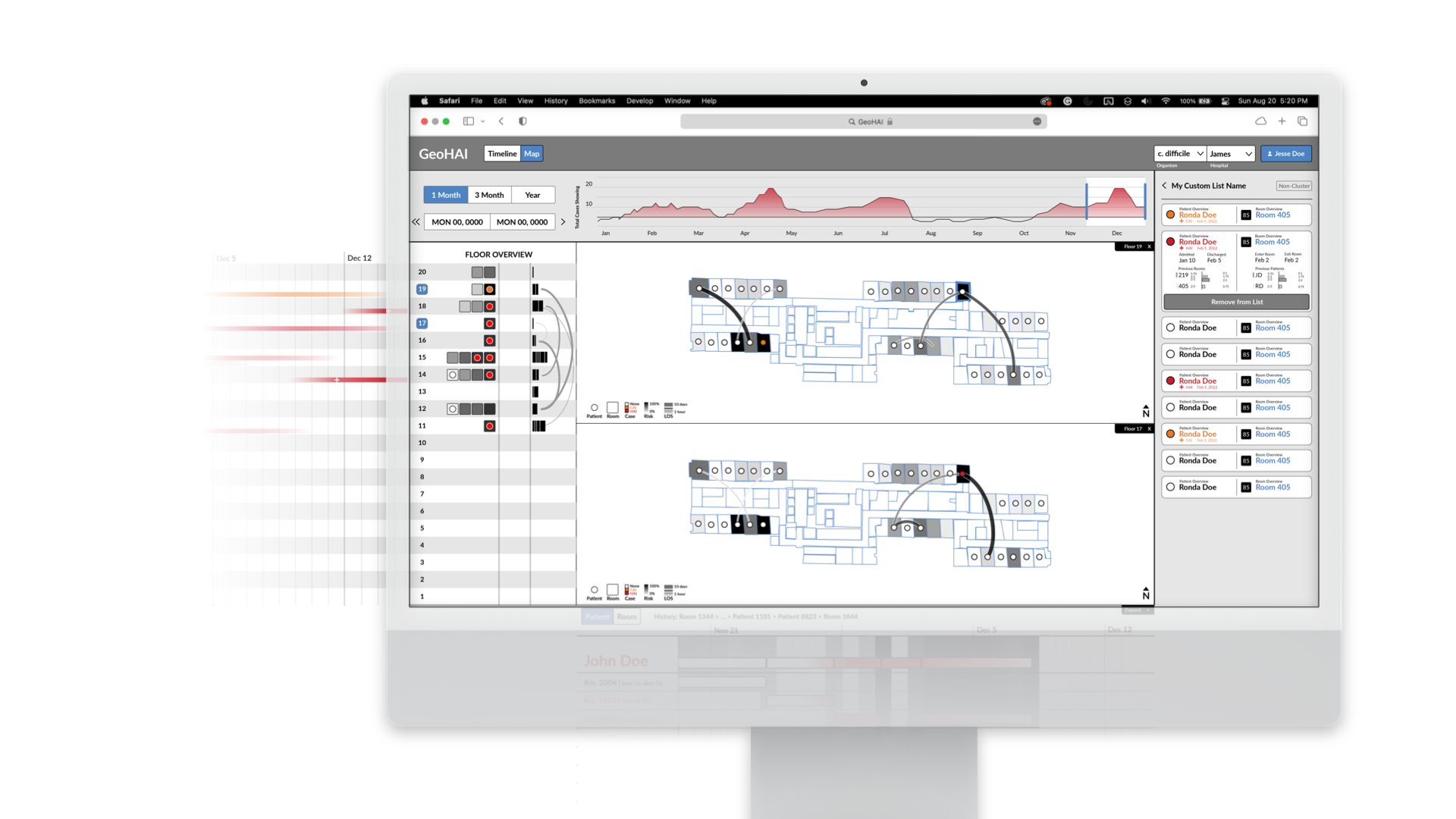